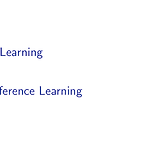
Lecture 4 : Model-Free Prediction -Introduction -Monte-Carlo Learning -Blackjack Example -Incremental Monte-Carlo -Temporal-Difference Learning -Driving Home Example -Random Walk Example -Batch MC and TD -Unified View -TD(λ) -n-Step TD -Forward View of TD(λ) -Backward View of TD(λ) -Relationship Between Forward and Backward TD -Forward and Backward Equivalence Model-Free Reinforcement Learning M..
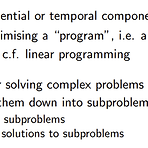
Lecture 3 : Planning by Dynamic Programming -Introduction -Policy Evaluation -Iterative Policy Evaluation -Example: Small Gridworld -Policy Iteration -Example: Jack's Car Rental -Policy Improvement -Extensions to Policy Iteration -Value Iteration -Value Iteration in MDPs -Summary of DP Algorithms -Extensions to Dynamic Programming -Asynchronous Dynamic Programming -Full-width and sample backups ..
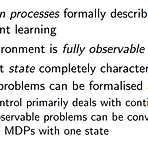
Lecture 2 : Markov Decision Process -Markov Processes -Introduction -Markov Property -Markov Chains -MRP -Markov Reward Processes -MRP -Return -Value Function -Bellman Equation -Markov Decision Processes -MDP -Policies -Value Functions -Bellman Expectation Equation -Optimal Value Functions -Bellman Optimality Equation Introduction to MDPs 전에 state에서 배운 내용의 연장선입니다. 거의 모든 강화학습의 문제는 MDP로 만들 수 있습니다...
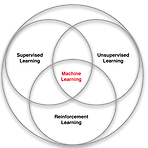
구글 딥마인드의 David Silver 교수님의 수업 자료를 바탕으로 정리한 RL강의입니다. 목차에 하나씩 추가하며 진행할 계획입니다. Lecture 1 : Introduction to Reinforcement Learning -About RL -The RL Problem -Reward -Environment -State -Inside An RL Agent -Problems within RL Machine Learning 기계학습에는 supervised learning, unsupervised learning, reinforcement learning 세가지가 존재합니다. 밑에 그림에서 공집합의 결과가 ML로 되있는 것처럼 보이는데, 사실 전체 큰 원이 ML이라고 보시면 됩니다. 각자 학습 방법에는 공통..